Home > Company News > Pftl101a 1.0kn 3bse004166r1 horizontal tension sensor
Company News
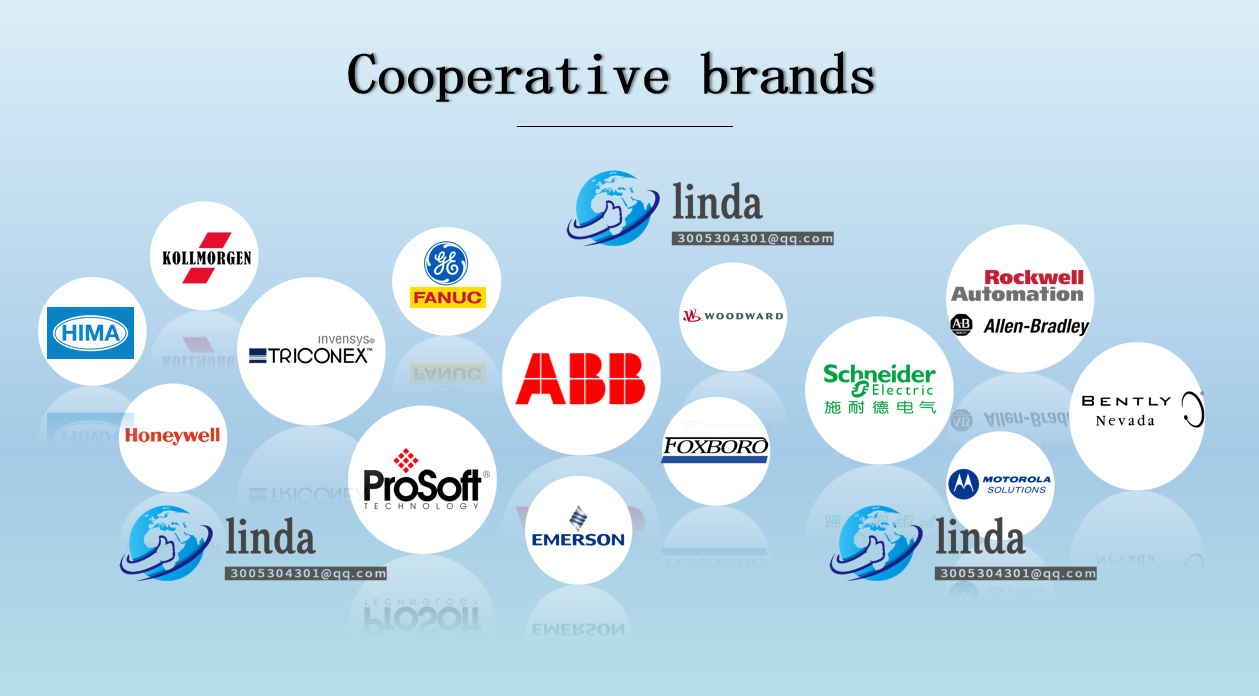
Pftl101a 1.0kn 3bse004166r1 horizontal tension sensor
Email: 3005304301@qq.com
Mobile: 86 18050025541
Whatsapp/ Wechat/Skype: 18050025541
Sales Manager: Linda
ABB PFT series tension sensors pftl101b 2.0kn, pftl101a 1.0kn, pftl101a 1.0kn 3bse004166r1, pftl101a-2.0kn 3bse004172r1, pftl101a 1.0kn 3bse004166r1, pftl101a 2.0kn 3bse004172r1, pftl101b 2.0kn, pftl101b 20KN 3bse004203r1, pftl101b 5.0kn 3bse004191r1, pftl101be 2.0kn 3bse04214r1, pftl101b 201c 10kN 3bse007913r0010, pftl301e 1.0 Knabb fetch and output instructions (LD / LDI / LDP / LDF / out)
In March 2016, the match between alphago and professional go players aroused people's high attention to artificial intelligence. Is it the intelligence of human brain that the computer has defeated the top player of human beings in a recognized very complex calculation and intelligence task? From the perspective of system structure, alphago combines deep neural network training with Monte Carlo simulation [1]. In a broad sense, the deep neural network is a brain like computing form, and the Monte Carlo method is to give play to the advantages of the machine's computing speed and simulate a large number of possibilities for further judgment. Now it seems that this is not the brain's working mechanism. Therefore, alphago can be said to be a success achieved by combining the computing and intelligence of brain like and non brain like, and giving full play to their respective strengths. In addition to the deep neural network used by alphago, what other aspects of brain like computing and intelligence are being studied? What kind of breakthroughs may be brought about in the near future?
1. Rich interfaces, supporting Ethernet, serial port, can port, IO port and other equipment access and Ethernet, 2G / 3G / 4G all network access;
2. Hundreds of industrial protocols are embedded, supporting more than 99% PLC and most industrial equipment access;
3. 8GB local storage SD card support, support local data cache and offline application;
4. Three in one serial port, supporting RS485 / RS232 / RS422 electrical interfaces;
5. Support edge computing, realize data optimization, real-time response, agile connection, model analysis and other services at the edge nodes of the Internet of things, effectively share cloud computing resources and support simultaneous access of multiple devices;
6. Support dc9 ~ 36V wide voltage input, adapt to a variety of complex industrial sites;
7. Support LED lamp customization, and users can define LED lamps as required (such as equipment status, edge computing results, etc.);
8. No client, support remote upload and download of on-demand connection, and effectively save network traffic;
9. Support gateway health self diagnosis, and quickly detect gateway faults;
10. Support multiple standard VPNs (PPTP / L2TP / IPSec / OpenVPN);
11. Support network active / standby mode, and intelligently switch network access mode according to network conditions (support intelligent network diagnosis);
12. Powerful cloud software center support, which can install corresponding firmware and applications according to actual application scenarios;
13. It supports multiple remote control modes (no password / password / disabled), and has physical remote control switch and one key switch remote control function;
14. Support multi link well development data acquisition;
15. Support 4G flow detail analysis and flow control;
16. Support gateway remote management; Support network self recovery;
17. Support the hybrid positioning mode of the base station and GPS and the GPS location presentation of the local web end;
18. Support local web endpoint table configuration, support local configuration design and presentation;
19. Industrial level edge computing gateway, with maximum data collection support of 5000 points;
20. Support data multiplexing and third-party platform access. Generally speaking, brain like computing refers to making essential changes to the existing computing system and system at the levels of hardware implementation and software algorithm by referring to the basic rules of information processing in the brain, so as to achieve substantial improvements in computing energy consumption, computing capacity and computing efficiency. In the past few decades, the rapid development of communication and computer technology has brought about the information revolution. However, the existing computing systems still face two serious development bottlenecks: first, the system energy consumption is too high; second, the processing capacity of cognitive tasks (such as understanding language and complex scenes) that the human brain can easily handle is insufficient, and it is difficult to support high-level intelligence. The obvious advantages of the brain in these two aspects make it a very promising direction to learn from the brain. Brain like computing is a high degree of intersection and integration of life science, especially brain science and information technology. Its technical connotation includes a deep understanding of brain information processing principles, and on this basis, a new processor, algorithm and system integration architecture are developed and applied to a new generation of artificial intelligence, big data processing, human-computer interaction and other fields. Brain like computing technology is expected to make artificial information processing system produce intelligence comparable to human brain with very low energy consumption. Many people believe that the substantive progress in this direction will really open the prelude to the intelligent revolution, thus bringing profound changes to social production and life. [2] the research on brain like computing can be roughly divided into three aspects: the research on neuroscience, especially the basic principles of brain information processing, the research on brain like computing devices (hardware) and the research on brain like learning and processing algorithms (software). In the field of neuroscience, in the past few decades, especially in the past 10 years or so, very rapid development has been achieved. Now we have accumulated a wealth of knowledge about the working principle of the brain, which provides an important biological basis for the development of brain like computing. The human brain is a complex network composed of nearly 100 billion neurons through hundreds of trillion contact sites (synapses). The realization of various brain functions such as sensation, movement and cognition is based on the orderly transmission and processing of information in this huge network. Through the efforts of several generations of neuroscientists, the structure and function of individual neurons have been better understood. However, there are still many problems to be solved as to how neurons with relatively simple functions can be organized through networks to form the most efficient information processing system we know now. At the micro level, the brain network is represented by the connections of neural synapses, at the mesoscopic level, by the connections of individual neurons, and at the macro level, by the connections of brain regions and sub regions. The information processing on different scales of brain networks has important differences and is closely related to each other, which is a unified whole. At present, the research hotspot of neuroscience mainly focuses on analyzing the structure of brain network at the above levels, observing the activities of brain network, and finally clarifying the function of brain network, that is, the mechanism of information storage, transmission and processing. To achieve this goal, the key technologies that need to be broken through are accurate and rapid determination of brain network structure, large-scale detection and regulation of brain network activities, and efficient analysis of these massive data. In addition, it is also urgent to establish appropriate models and theories under the constraint of experimental data to form a complete understanding of brain information processing [3]. The original intention of the research on brain like computing devices is to greatly reduce the power consumption without affecting the performance, or greatly improve the speed under similar power consumption. Although modern computers have amazing computing power and speed, it is accompanied by high energy consumption. The power consumption of large-scale computers is often on the order of megawatts, compared with that of the adult brain, which is only about 20 W. The huge energy consumption seriously restricts the further development of the system in the direction of miniaturization (because it is difficult to dissipate heat), and also makes complex embedded applications and remote applications, such as aerospace exploration, lack sufficient computing capacity support (because it is difficult to carry enough energy). An important reason for the high energy consumption of modern computers is the widespread use of Feng Neumann architecture. In the Feng architecture, the information processing unit and the storage unit are separated, so in the process of operation, it is necessary to transfer data between the processing unit and the storage unit frequently. This seemingly simple process can contribute nearly 50% of the power consumption of the system. In contrast, in the biological brain, the processing of information is realized in the neural network, while the data itself is distributed and stored in various nodes of the network (such as the ion concentration in neurons) and the connections between nodes (such as the strength of synapses). The operation and storage are highly integrated in structure. Thus, it has become a very attractive direction to simulate the function of a single neuron with a small number or even a single electronic device, and to form a large-scale parallel processing network in a brain like manner for computing. At present, the research focuses include finding more suitable devices to simulate a single neuron (such as memristor), and designing processors based on non Feng's system. Recently, the truenoth chip developed by IBM is a representative development in this field. Due to the use of non Feng architecture and a series of other measures, the power consumption is reduced by nearly 2 orders of magnitude (Figure 1) [4]. Other important developments include the development of special processors, which are specially optimized for brain like algorithms such as deep neural networks to improve speed and reduce power consumption [5]. Since the algorithms in this field have been mature in image and speech recognition, such special processors are expected to be put into practical use earlier. ABB PFT series tension sensors pftl101b 2.0kn, pftl101a 1.0kn, pftl101a 1.0kn 3bse004166r1, pftl101a-2.0kn 3bse004172r1, pftl101a 1.0kn 3bse004166r1, pftl101a 2.0kn 3bse004172r1, pftl101b 2.0kn, pftl101b 20KN 3bse004203r1, pftl101b 5.0kn 3bse004191r1, pftl101be 2.0kn 3bse04214r1, pftl101b 201c 10kN 3bse007913r0010, pftl301e 1.0 kn
Brain like processors that can greatly reduce energy consumption or speed up speed will undoubtedly help to achieve a higher level of intelligence. However, in order to truly realize human like general artificial intelligence, in addition to such hardware foundation, the key is to understand the calculation of biological brain for information, that is, brain like processing and learning algorithms. For this research direction, a common concern is: the understanding of the working mechanism of the brain in neuroscience is far from enough. Can we carry out effective brain like algorithm research? In this regard, we can get some enlightenment from the widely successful deep neural network. From many aspects such as the connection mode of neurons and the training rules, the deep neural network is still far from the real brain network. However, it essentially draws on the multi-layer structure of the brain network (the source of the word "depth"), and the multi-layer and step-by-step processing structure of the brain, especially the visual pathway, is the basic knowledge acquired in neuroscience. This shows that we do not need to fully understand the working principle of the brain before we can study brain like algorithms. On the contrary, what is really enlightening is probably the relatively basic principle. Some of these principles may have been known by brain scientists, while others may still be discovered. The elucidation of each basic principle and its successful application in artificial information processing systems may bring about large or small progress in brain like computing research. It is very important that this process of continuous discovery and transformation can not only promote the progress of artificial intelligence, but also simultaneously deepen our understanding of why the brain can process information so efficiently [6], thus forming a virtuous circle in which brain science and artificial intelligence technology promote each other.